Unlocking the Power of Labeled Image Datasets for Your Business
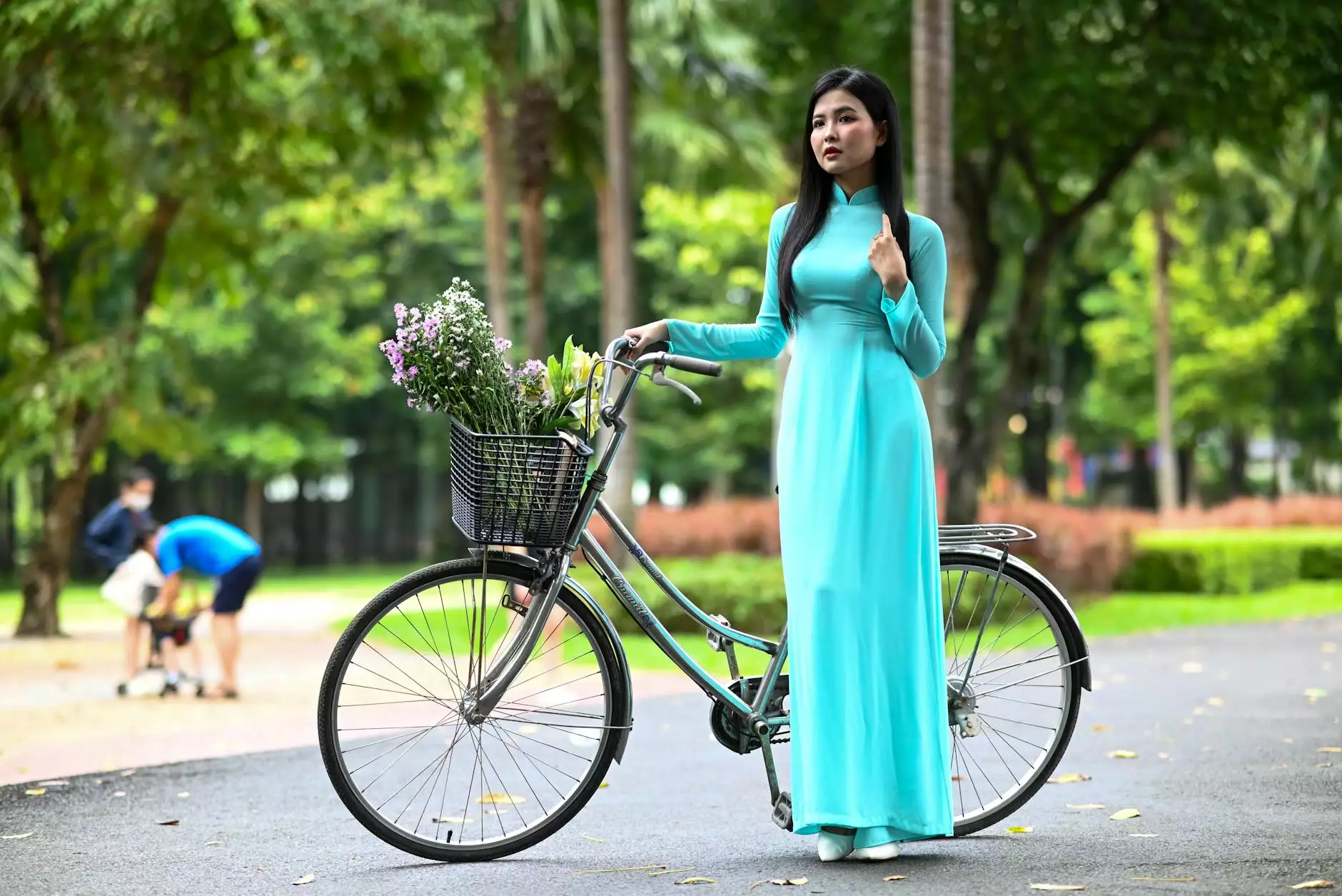
The modern business landscape is increasingly driven by data, and the emergence of artificial intelligence (AI) and machine learning (ML) has only intensified this trend. One of the pivotal components in enabling successful AI applications is the use of labeled image datasets. These datasets provide the necessary data that AI models need to learn, adapt, and make informed decisions that can lead to greater operational efficiency and competitive advantage.
What Are Labeled Image Datasets?
Labeled image datasets are collections of images that have been annotated with specific labels, often identifying objects, features, or classifications within the images. This annotation serves as training data for machine learning algorithms, facilitating their understanding and ability to recognize similar patterns in new, unannotated images.
The Importance of Labeled Image Datasets
In the sphere of AI, labeled image datasets are essential for numerous reasons:
- Model Training: They allow algorithms to learn from examples, improving accuracy and performance.
- Object Recognition: Essential for tasks such as facial recognition, autonomous driving, and medical imaging.
- Quality Assurance: High-quality labels lead to more reliable AI outputs.
- Data Diversity: Comprehensive datasets ensure that models can generalize well across different conditions and scenarios.
The Role of Data Annotation Tools
At the core of creating labeled image datasets lies a robust data annotation tool. Businesses utilizing data annotation tools can efficiently manage the complexity of labeling large volumes of images. At KeyLabs.AI, we provide advanced data annotation platforms that streamline the process, ensuring quality and consistency in annotations.
Key Features of Our Data Annotation Tools
Our data annotation tools are designed with user experience and efficiency in mind:
- User-Friendly Interface: Simplifies the annotation process, allowing teams to focus on accuracy.
- Multiple Annotation Types: Supports bounding boxes, segmentation, and keypoint annotations for comprehensive labeling.
- Collaboration Features: Facilitate teamwork among annotators, improving productivity.
- Quality Control Mechanisms: Built-in validation and review systems to ensure high-quality outputs.
Building a Labeled Image Dataset: Best Practices
Creating an effective labeled image dataset involves strategic planning and execution. Here are some best practices for businesses:
1. Define the Purpose of Your Dataset
Identifying the specific application of your dataset is crucial. Whether for object detection in autonomous vehicles or medical diagnosis, clarity on your goal will guide your annotation efforts.
2. Collect Diverse Data
To ensure that your AI model can generalize and perform well, it is important to collect a diverse set of images that represents various scenarios, lighting conditions, and backgrounds.
3. Use Quality Annotation Tools
As mentioned earlier, leveraging robust data annotation tools can significantly affect the quality of your labeled image datasets. Choose tools that offer scalability, flexibility, and ease of use.
4. Ensure Accurate and Consistent Labeling
Inaccuracy in labeling can lead to poor model performance. It is essential to implement strong quality control measures to check for consistency and accuracy in annotations throughout the dataset.
5. Continuously Update Your Dataset
As new data becomes available or as the environment changes, continuously updating your labeled image datasets is critical to maintain the relevance and accuracy of the AI models.
Applications of Labeled Image Datasets Across Industries
Labeled image datasets are utilized across various industries, revolutionizing how businesses operate:
1. Healthcare
In medical imaging, labeled datasets assist in training models that can detect and diagnose diseases from images, such as identifying tumors in radiology scans.
2. Autonomous Vehicles
Self-driving cars rely heavily on labeled image datasets for object detection, identifying pedestrians, road signs, and other vehicles.
3. Retail and E-commerce
Businesses in retail utilize labeled image datasets for visual search capabilities, allowing consumers to search for products visually, enhancing the shopping experience.
4. Security and Surveillance
Facial recognition systems rely on extensive labeled image datasets for identification purposes in security applications.
How KeyLabs.AI Can Elevate Your Data Strategy
By harnessing the capabilities of our data annotation platform, businesses can create high-quality labeled image datasets that drive innovation and efficiency in their operations. Here’s how KeyLabs.AI offers unparalleled support:
Custom Annotation Services
We provide tailored annotation services that meet your particular needs, ensuring that your datasets are created accurately and efficiently.
Scalable Solutions
Our platform is built to scale, accommodating projects of any size, from small sets of images to massive datasets.
Expert Annotators
KeyLabs.AI employs skilled annotators who are trained to maintain high-quality standards in annotation, leading to reliable datasets.
Conclusion: The Path Forward with Labeled Image Datasets
In conclusion, the importance of labeled image datasets cannot be overstated. They form the backbone of AI and ML applications across numerous industries, driving growth, efficiency, and innovation. By utilizing advanced data annotation tools such as those offered by KeyLabs.AI, businesses can ensure they have the quality data they need to succeed in a data-driven future.
As the demand for AI solutions grows, so does the need for high-quality labeled image datasets. Take the first step towards transforming your business today by exploring how KeyLabs.AI can assist you in achieving your data annotation goals.